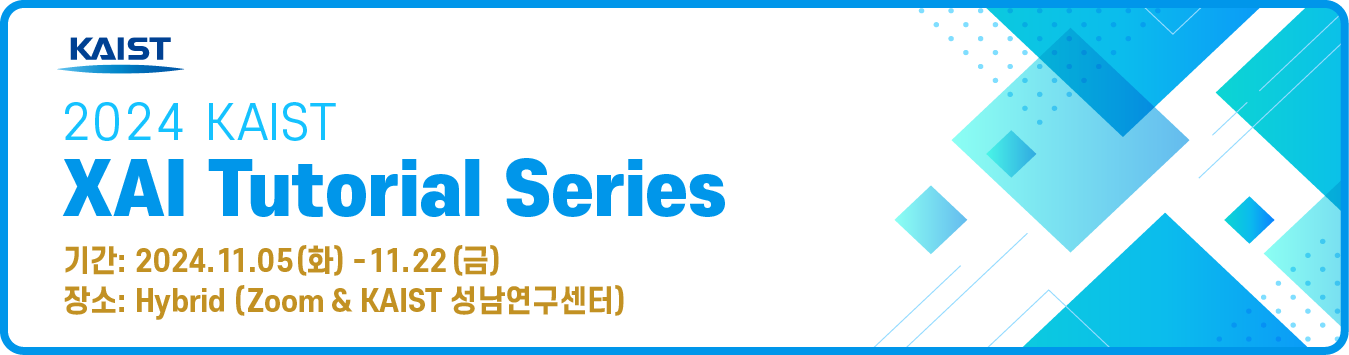
Introduction
최근 신뢰성 있는 AI 활용을 위한 규제와 입법이 본격화됨에 따라 AI 모델의 설명가능성 기술에 대한 관심이 그 어느 때보다 높아지고 있습니다. 이에 국내 최대의 설명가능 인공지능(XAI) 연구자 그룹인 KAIST 설명가능 인공지능연구센터(공동주최 성남연구센터)에서 2024 XAI 튜토리얼 시리즈를 개최합니다. 올해 프로그램은 XAI의 주요 알고리즘부터 최신 연구 주제까지 총 7일간 16개 발표세션으로 구성하였습니다. 많은 관심과 참여 부탁드립니다! KKAIST XAI Research Center (co-hosted by KAIST Kim Jaechul Graduate School of AI Seongnam Research Center) will host an XAI Tutorial Series November, 2024. KAIST XAI Research Center will host an XAI Tutorial Series 2024 from Nov. 5th to Nov. 22nd. This Tutorial Series aims to bring together both AI researchers and industrial practitionersto learn XAI (Explainable AI) from the fundamentals to its current topics. On DAY7, we organized three invited lectures on AI regulatory landscapes and the latest research topics in concept-based explanations.
Target audience: Researchers, Industry practitioners, and anyone interested in the XAI
Schedule: 2024.11.05(Tue)~11.22(Fri) 15:00-17:00 | Day7(Fri) 16:00-18:00
Location: Hybrid (KAIST Seongnam Research Center 18F, Online), Zoom link will be provided on registration.
Presentation: 50min
Language: Korean, Session 15 & 16 will be in English.
Program
1. 설명 가능한 인공지능의 최신 동향 (Recent Trends in Explainable Artificial Intelligence)
Jaesik Choi (Professor, KAIST AI)
Abstract:
최근 심층신경망과 같은 복잡한 AI기술이 군사, 금융, 인사, 자율주행 등 미션 크리티컬한 분야에 널리 활용되고 있습니다. 장애나 오류가 발생하면 사용자와 비즈니스에 심각한 영향을 끼치게 되는 이러한 응용에서는 AI기술을 안전하게 사용하는 것이 무엇보다도 중요합니다. 이 강연에서는 안전하고 신뢰할 수 있는 AI시스템을 구현하는 데에 필수요소인 설명가능한 인공 지능의 원리와 최근 동향을 살펴봅니다.
As complex artificial intelligence (AI) systems such as deep neural networks is used for many mission critical task such as military, finance, human resources and autonomous driving, it is important to secure the safe use of such complex AI systems. In this talk, we will overview the principles and recent advances in explainable artificial intelligence.
Speaker Bio:
2019~현재 KAIST 김재철AI대학원 교수/(주)인이지 대표이사
2019~현재 KAIST 설명가능 인공지능연구센터 센터장
2013-2019 UNIST 전기전자컴퓨터공학부 교수
2013 미국 Lawrence-Berkeley 연구소 박사후연구원
2012 미국 일리노이 주립대학교 박사졸업
연구분야: 설명가능 인공지능, 시계열데이터 예측, 기계학습/딥러닝
2019~present Professor, KAIST Kim Jaechul Graduate School of AI
2019~present CEO, INEEJI Inc.
2013~2019, Professor, Dept. of Electrical and Computer Engineering, UNIST
2013 Post-doc Researcher, Lawrence Berkeley National Laboratory
2012 Ph.D. University of Illinois (Urbana-Champaign)
Research Topics: Explainable AI, Learning and Inference for Time Series Data(Large-scale), Deep Learning Applicatons
2. 지역적 설명 방법 (XAI Methods I: Local Explanation Methods)
Myeongjin Lee (SAILab, KAIST AI)
Abstract:
지역적 설명 방법은 모델의 개별 예측에 대한 설명을 제공합니다. 본 발표에서는, 여러 방법론들 중, 개별 입력 데이터의 여러 특징들이 해당 예측에 얼마나 기여했는지를 계산하여 설명을 제공하는 방법에 대해 소개합니다. 구체적으로, 모델의 종류에 상관없이 적용할 수 있는 (1) 모델 불가지론적 (model-agnostic) 방법과 (2) 딥러닝 모델에 특화된 (model-specific) 방법에 관한 대표적인 방법들을 소개합니다.
Local explanation methods provide explanations for individual predictions of a model. This talk introduces methods that quantify how different features of individual input data contribute to the corresponding prediction. Specifically, we will present representative methods from two categories: (1) model-agnostic methods, which can be applied regardless of the model type, and (2) model-specific methods, which are tailored to deep learning models.
Speaker Bio:
KAIST AI 대학원 석사 과정 학생으로 최재식 교수님의 지도를 받고 있습니다. 현재는 diffusion model의 메커니즘을 설명하기 위해 diffusion model의 내부 모듈들을 분석하는 연구에 집중하고 있습니다.
Myeongjin Lee is a M.S. student at the Graduate School of AI, KAIST, under the supervision of Prof. Jaesik Choi. His current research focuses on analyzing internal modules of diffusion models to explain their internal mechanisms.
3. 전역적 설명 방법 (XAI Methods II: Global Explanation Methods)
Youngju Joung (SAILab, KAIST AI)
Abstract:
전역적 설명 방법은 머신러닝 및 딥러닝 모델이 산출하는 모든 예측 결과를 설명하는 방식입니다. 이 접근법은 모델의 전반적인 행동에 대한 통찰을 제공하여, 일반적인 메커니즘을 이해하거나 모델을 디버깅할 때 특히 유용합니다. 본 발표에서는 전역적 설명 방법을 고전적인 모델 불가지론적 방법(model-agnostic method), 프로토타입 기반 방법(prototype-based method), 그리고 개념 기반 방법(concept-based method)으로 구분하여 소개하고자 합니다.
Global explanation methods aim to interpret all prediction outcomes generated by machine learning and deep learning models. These approaches provide insights into the overall behavior of the model, making them especially useful for understanding general mechanisms or for debugging. In this presentation, we will introduce global explanation methods by categorizing them into classical model-agnostic methods, prototype-based methods, and concept-based methods.
Speaker Bio:
KAIST 인공지능 대학원에 재학중인 석사 과정 학생입니다. 최재식 교수님이 지도하시는 SAIL 연구실에서 설명가능인공지능 기술에 대해 연구하고 있습니다. 현재 심층 신경망의 vulnerability를 linear region의 관점에서 설명하고, 이를 기반으로 robust한 모델을 만들기 위한 방법에 대해 연구하고 있습니다.
Youngju Joung is a master’s student at the Graduate School of AI at KAIST, advised by Professor Jaesik Choi in the SAIL Lab. She is conducting research on explainable AI, focusing on explaining the vulnerability of deep neural networks from the perspective of linear regions and developing methods to create robust models based on this understanding.
4. XAI의 평가지표 (XAI Evaluation)
Sol A Kim (SAILab, KAIST AI)
Abstract:
예측 모델의 성능을 평가하기 위해 표준 평가 지표가 존재하는 반면, 설명 가능 인공지능 (Explainable AI, XAI)에 대해 합의된 평가 지표는 없습니다. 그 결과, XAI에 대한 일반적인 평가 방법은 시각적 사례를 제시하거나 목적에 따른 평가 함수를 선택하여 검증하는 것입니다. 본 발표에서는 사용자의 목적에 따라 평가 방법을 선택할 수 있도록 다양한 평가 방식을 분류하여 소개합니다.
While standard evaluation metrics exist to assess the performance of predictive models, there are no agreed-upon evaluation metrics for Explainable AI (XAI). As a result, common evaluation methods for XAI involve presenting visual examples or selecting evaluation functions based on specific objectives. In this presentation, we introduce a classification of various evaluation methods to help users select appropriate approaches based on their configurations.
Speaker Bio:
KAIST AI 대학원의 석박통합과정을 밟고 있습니다. SAIL 연구실의 최재식교수님의 지도하에 설명가능 인공지능을 통한 AI모델의 편향 완화에 대한 연구를 하고 있습니다.
Sol A Kim is a Ph.D candidate at the KAIST Graduate School of AI. Under the supervision of Professor Jaesik Choi from the SAIL Lab, she has been conducting research on mitigating bias in AI models through explainable AI.
5. 언어모델의 설명 알고리즘 개요와 평가 방안 (Explaining Language Models: Key Methods and Evaluations)
Nari Kim (Research Professor, KAIST XAI Center)
Abstract:
거대 언어모델(LLM)이 뛰어난 성능을 보여줌에 따라 다양한 응용분야로 빠르게 확산되고 있습니다. 그러나 거대 언어모델의 복잡성과 고차원 내부 표현으로 인해 이러한 모델의 작동 방식을 설명하는 것은 큰 도전이 되고 있습니다. 언어모델이 어떻게 작동하는지를 명확히 이해하는 것은 언어모델의 한계를 인식하고 안전하게 활용하기 위한 선결과제이기 때문입니다. 본 발표에서는 언어모델을 설명하기 위한 주요 접근방식을 소개하고 이를 판별 모델 (Discriminative Language Models) 설명에 적용한 예를 살펴봅니다.
The impressive performance of large language models (LLMs) is garnering attention and is quickly being applied to various areas. However, a significant challenge for researchers is the lack of transparency stemming from these models' structural complexity and high-dimensional internal representations. To use language models safely, it is crucial to understand their inner workings and acknowledge their limitations. This presentation introduces essential methods for explaining language models and provides several explanation examples.
Speaker Bio:
통계적 방법을 활용한 자연어 처리 모호성 해결에 대한 연구로 서울대학교 컴퓨터공학과에서 박사학위를 받았습니다. 기업에서 개발자와 사업 담당으로 커리어를 쌓으며 기계번역, 소셜 미디어 분석, 텍스트마이닝 등 다양한 제품의 개발과 사업 리더로 일했습니다. 2022년부터 KAIST에서 연구교수로 일하며 여러 연구자들과 함께 설명 가능하고 신뢰할 수 있는 AI 시스템 연구를 하고 있습니다.
She received her Ph.D. in Computer Engineering at Seoul National University with a thesis on ambiguity resolution in natural language processing using corpus-based statistical methods. She began her career as a developer and later became a business director, where she contributed to a range of products, including machine translation, text mining, and social media analytics. Since 2022, she has been a research professor at KAIST, leading a team of researchers to develop explainable and trustworthy AI systems.
6. 생성 언어모델의 설명: 프롬프팅 기반 설명 (Explaining Generative LLMs: Prompting-based Explanations)
Cheongwoong Kang (SAILab, KAIST AI)
Abstract:
본 발표에서는 프롬프팅 기반 기법을 사용하여 생성 대형 언어 모델(LLM)의 출력을 설명하는 접근 방식을 탐구합니다. 모델이 입력과 상호작용하는 방식을 분석하여, 출력 결정에 기여하는 입력의 중요한 부분을 밝혀내고, 모델의 추론 과정을 읽기 쉽게 설명하며, 입력 요소들이 출력에 어떻게 연결되는지 구조화된 해석을 제공합니다.
This presentation explores approaches to explaining the outputs of generative large language models (LLMs) using prompting-based techniques. By analyzing how the model interacts with inputs, we aim to uncover the most relevant parts of the input that contribute to its decisions, provide readable explanations for its reasoning, and present structured interpretations to clarify how various components of the input lead to specific outputs.
Speaker Bio:
강청웅은 KAIST 인공지능 대학원에서 박사 과정을 밟고 있으며, 최재식 교수님이 이끄는 SAIL 연구 그룹에 소속되어 있습니다. 그의 연구는 언어 모델에 내재된 지식을 검증하고, 이러한 지식을 제어하거나 확장하여 모델을 개선하는 데 중점을 두고 있습니다.
Cheongwoong Kang is a PhD candidate at the Graduate School of AI, KAIST, affiliated with the SAIL led by Professor Jaesik Choi. His research focuses on verifying knowledge embedded within language models and improving them by controlling or augmenting their knowledge.
7. 생성모델과 XAI (Generative Models and XAI)
Junho Choi (SAILab, KAIST AI)
Abstract:
생성 모델은 목표 분포를 모방하는 모델로, 새로운 이미지, 문장, 사운드 등 다양한 데이터를 생성하는 데 사용할 수 있다. XAI에서 생성 모델은 다른 모델을 설명하는 프로세스의 일부인 설명 모델, 또는 설명할 대상인 대상 모델로 사용된다. 이 프레젠테이션에서는 이미지 영역에서 두 경우에 대한 몇 가지 예를 간략하게 소개한다.
Generative models are models that mimic a target distribution. They are used for creating new instances that belong to the distribution, such as new images, sentences, or sounds. In XAI, these models are used as explainer or explainee, acting as a part of the process to explain another model or as the target model to be explained. This presentation introduces several examples for both cases in the image domain, discussing a general overview of the algorithms.
Speaker Bio:
최준호는 KAIST 김재철 AI대학원에서 공부하고 있는 석박통합과정 학생으로 SAIL연구실의 최재식 교수 밑에서 공부하고 있다. 그는 섭동을 사용하는 입력기여도 분석 방법의 개선을 연구 목표로 삼고 있다.
Junho Choi is a MS/PhD student at Kim Jaechul Graduate School of AI at KAIST. He is also a member of Statistical Artificial Intelligence Lab, studying under Professor Jaesik Choi. His research interests include improving feature attribution methods that use perturbations.
8. 확산모델 기반의 이미지 생성 AI에 대한 설명 (Understanding Diffusion-based Generative Models)
Dahee Kwon (SAILab, KAIST AI)
Abstract:
이번 발표에서는 최근 주목받고 있는 텍스트 기반 이미지 생성 모델, 특히 확산 모델을 설명하고 수정하는 방법들을 소개합니다. 확산 모델이 내부적으로 학습한 다양한 특징(feature)과 각 모듈의 역할을 분석하고, 이를 수정하여 더 나은 이미지 생성을 이끌어내는 방법을 탐구하고자 합니다. 또한, 기존의 전통적인 설명 가능한 인공지능(XAI) 기법들이 확산 모델에 어떻게 적용될 수 있는지를 살펴보며, 복잡한 확산 모델을 더 효과적으로 이해하는 방법도 함께 소개할 예정입니다.
This talk introduces text-to-image generation models that have recently gained attention, focusing particularly on diffusion models. We will explore the various features learned by diffusion models and analyze the roles of different internal modules, aiming to understand how modifying these components can lead to better image generation. Additionally, we will examine how traditional explainable AI (XAI) techniques can be applied to diffusion models, offering insights into how we can better understand the complex diffusion models.
Speaker Bio:
9. 시계열 도메인 특화 XAI 기법 적용과 해석 (Domain-Specific XAI Techniques for Time Series)
Sehyun Lee (SAILab, KAIST AI)
Abstract:
이 발표에서는 시계열 데이터에 특화된 설명 가능한 인공지능(XAI) 기법을 다룬다. 특히 신경망에 적용된 시계열 데이터의 attribution 기법 예제를 통해, 이 기법이 어떻게 중요한 특징을 식별하는지 살펴보고, 해석 방법을 통해 시계열 모델의 의사결정을 더 투명하게 이해할 수 있는 방안을 논의한다. 또한, 시계열 신경망 모델에서 학습된 시간적 패턴의 prototype을 구하는 방법을 소개하고, 모델이 특정 시점에서 주목하는 패턴을 어떻게 분석할 수 있는지 설명한다.
This talk focuses on Explainable AI (XAI) techniques specifically designed for time series data. We'll explore how attribution methods can highlight key features in neural networks and discuss how to interpret these insights to better understand the decision-making process of time series models. We'll also introduce a technique for identifying prototypes of temporal patterns learned by these models and explain how to analyze the patterns that the model pays attention to at different points in time.
Speaker Bio:
이세현은 KAIST 김재철 AI 대학원에서 최재식 교수의 지도하에 박사 과정을 밟고 있습니다. 현재 연구는 딥러닝 모델의 자동화된 디버깅 기법과 다의미 뉴런을 분석하는 것을 중점적으로 다루고 있으며, AI 시스템의 해석 가능성과 기능성을 향상시키는 것을 목표로 하고 있습니다.
Sehyun Lee is a PhD candidate at the Kim Jaechul Graduate School of AI, KAIST, under the supervision of Professor Jeasik Choi. She is dedicated to advancing the field of machine learning, with a focus on developing automated debugging techniques for deep learning models. At the forefront of exploring polysemantic neurons and their applications, her work aims to enhance the interpretability and functionality of AI systems in complex environments.
10. XAI를 위한 인과관계 (Basics of Causality for XAI)
Won Jo (SAILab, KAIST AI)
Abstract:
Causality는 변수 간의 단순한 상관관계를 넘어, 원인과 결과의 방향성을 명확하게 구분할 수 있도록 해줍니다. 이를 통해 모델 결정의 근거를 명확히 밝혀낼 수 있고, 특정 입력과 특징이 결정에 미친 영향을 보다 정확하게 설명할 수 있습니다. 본 발표에서는 설명가능성을 높이기 위한 Causality의 기본 개념을 소개합니다. 또한, Causality를 통해 모델 결정을 설명한 최근 연구를 바탕으로, XAI에서 Causality를 활용하는 방법에 대해서도 다룰 예정입니다.
Causality goes beyond correlations between variables, enabling us to clearly identify the direction of cause and effect. This allows us to more precisely describe the reasons behind model decisions and to provide a more detailed explanation of the ways that specific inputs and features affect those decisions. This talk introduces the basic concepts of causality to improve explainability. Furthermore, recent works that use causality to explain model decisions will be summarized. This will provide the application of causality in XAI.
Speaker Bio:
조원은 KAIST 김재철AI대학원에서 박사 과정을 밟고 있으며, SAI 연구실에서 최재식 교수님의 지도를 받고 있습니다. 현재 그의 연구는 모델 의사결정 과정 내 인과성을 분석함으로써 모델 내부에서 근거를 찾는 것에 중점을 두고 있습니다.
Won Jo is a Ph.D. student at the Statistical Artificial Intelligence Lab (SAILab), led by Prof. Jaesik Choi, at KAIST. His current research focuses on analyzing causality within the model's decision-making process to identify internal reason.
11. Plug-and-Play XAI Framework 소개 (Introduction to Plug-and-Play XAI Framework)
Chanwoo Lee (SAILab, KAIST AI)
Abstract:
이 세션에서는 주어진 모델과 데이터를 대상으로 설명가능인공지능(XAI, Explainable Artificial Intelligence) 기술을 보다 쉽게 적용할 수 있도록 돕는 PnP(Plug-and-Play) XAI Framework를 소개합니다. 먼저, 기존의 다양한 XAI 프레임워크들의 장단점을 비교하고, Plug-and-Play 방식을 채택한 PnP XAI Framework의 필요성을 논의합니다. 특히, 산업 현장에서 XAI 기술이 성공적으로 도입되기 위해서는 XAI에 대한 깊은 지식이 없는 개발자들도 쉽게 적용할 수 있어야 하며, 이를 가능하게 하는 PnP XAI Framework의 역할을 강조합니다.
In this session, we introduce the PnP (Plug-and-Play) XAI Framework, which helps apply Explainable Artificial Intelligence (XAI) technology to given models and data more easily. First, we compare the advantages and disadvantages of various existing XAI frameworks and discuss the necessity of the PnP XAI Framework, which adopts the Plug-and-Play approach. In particular, for successful adoption of XAI technology in industrial settings, it must be accessible even to developers without in-depth knowledge of XAI. We emphasize the role of the PnP XAI Framework in making this possible.
Speaker Bio:
KAIST 김재철 AI대학원에서 최재식 교수님의 지도 하에 석사 과정을 밟고 있으며, "Plug and Play 방식의 XAI 적용 프레임워크 개발" 프로젝트와 관련된 연구를 진행하고 있습니다.
Chanwoo Lee is pursuing a Master's degree at the KAIST Graduate School of AI under the supervision of Prof. Jaesik Choi. His research is focused on the "Development of a Plug and Play XAI (Explainable AI) Application Framework" project.
12. Plug-and-Play XAI Framework 사용 튜토리얼 (Tutorials on Plug-and-Play XAI Framework)
Geonhyeong Kim (Researcher, KAIST XAI Center)
Abstract:
이 세션에서는 PnP XAI Framework의 기본 구조와 주요 API를 설명하여 프레임워크의 구성을 명확히 이해할 수 있도록 돕습니다. 마지막으로, 간단한 예제를 통해 실무적 관점에서 이 프레임워크가 산업 현장에서 어떻게 활용될 수 있는지를 쉽게 설명합니다.
In this session, we explain the basic structure and key APIs of the PnP XAI Framework to help users clearly understand how it is organized. Finally, we provide a simple example to illustrate how this framework can be practically utilized in industrial environments from a practical perspective.
Speaker Bio:
KAIST 설명가능 인공지능연구센터 연구원으로, PnPXAI Framework의 백엔드 개발을 담당하고 있습니다.
Geonhyeong Kim is a researcher at the KAIST XAI Center. He is developing the backend for the PnPXAI Framework, an innovative framework that provides AI researchers and practitioners with advanced and user-friendly tools for explainable AI.
13. 메디컬 도메인에서의 XAI (XAI for Clinical Decision Support)
Jihyeon Seong (SAILab, KAIST AI)
Abstract:
메디컬 분야는 AI모델의 우수한 성능 뿐만 아니라, 그 예측의 원인을 설명하여 모델의 신뢰성을 보장하는 것이 중요합니다. 특히, 실제 병원에 AI를 적용하기 위해서는 우수한 성능과 환자별 맞춤으로 질병 원인을 설명하는 기술이 모두 필요합니다. 본 세션에서는 급성 신손상 (AKI) 질병에서 AI를 이용한 예측 및 환자별 맞춤 원인 설명 기술을 소개합니다. 먼저, AKI 예측 모델을 실제 임상 적용을 위한 개발 과정을 소개합니다. 다음으로, 환자별 맞춤으로 AI모델의 예측 원인을 설명하기 위한 설명 알고리즘 및 적용 예시를 소개합니다. 본 발표에서는 실제 임상 적용 사례를 통해 메디컬 분야에서의 설명성 활용 예를 살펴봅니다.
In the medical field, it is essential not only to achieve high performance in AI models but also to explain the reasons behind their predictions to ensure model reliability. Especially for applying AI in real hospitals, both high performance and the ability to explain disease causes tailored to individual patients are necessary. This session introduces prediction and patient-specific cause-explanation techniques using AI for Acute Kidney Injury (AKI). First, we present the development process of an AKI prediction model for practical clinical application. Next, we introduce explanation algorithms and application examples for explaining the prediction causes of AI models customized for each patient. Through actual clinical application cases, this presentation explores examples of utilizing explainability in the medical field.
Speaker Bio:
KAIST 김재철 AI대학원에서 최재식 교수님의 지도 하에 박사 과정을 밟고 있습니다. 시계열 및 메디컬 분야에 대해 연구하고 있으며, 분당서울대학교병원과 협업하는 프로젝트인 "급성신손상 예측 및 임상을 위한 설명성 제공" 연구를 진행하고 있습니다.
Jihyeon Seong is a PhD student at the KAIST Kim Jaechul Graduate School of AI under the supervision of Professor Jaesik Choi. Her research focuses on the fields of time series and medical applications. Currently, she is working on a project titled 'Explainable Continuous Prediction of Acute Kidney Injury for Clinical Decision Support' in collaboration with Seoul National University of Bundang Hospital.
14. AI 규제 개요와 최신 동향 (Overview and Latest Trends in AI Regulation)
Kanghye Lee (Partner Attorney, 법무법인(유) 태평양)
Abstract:
2023년에는 생성형 AI 기술이 빠르게 확산되며 우리 사회에 큰 영향을 주었습니다. 2024년에도 AI 혁신이 계속될 것으로 예상되는 가운데, AI 기술의 안전한 활용과 신뢰성 확보를 위한 법적, 정책적 논의가 더욱 중요해지고 있습니다. 이번 세미나에서는 AI 관련 최근 입법 동향을 살펴보고, 신뢰성과 투명성을 강화할 수 있는 방안에 대해 함께 고민해보겠습니다.
In 2023, Generative AI technologies rapidly spread, bringing significant impact on our society. As we anticipate continued innovation in AI in 2024, the importance of legal and policy discussions on the safe use and trustworthiness of AI technologies is growing. In this seminar, we will explore recent legislative trends related to AI and discuss strategies to enhance trust and transparency.
Speaker Bio:
- 변호사, 법무법인(유한) 태평양(2021-현재)
- 연세대학교 법학전문대학원(2013)
- 연세대학교 법학과(2010)
- 금융보안원 가명·익명처리 적정성 평가위원(2024-현재)
- 강원가명정보활용지원센터 외부전문가위원(2021-현재)
- LG전자(2013-2021)
Slides Recording15. Understanding and Monitoring Model Behavior With Concept-based Explanations (English)
Maximilian Dreyer (Ph.D. Student, Fraunhofer HHI)
Abstract:
Concept-based explanations offer deep insights into neural networks, but analyzing individual explanations across large datasets can be inefficient. In this talk, we solve this by summarizing similar explanations with prototypes, providing a quick yet detailed overview of the model behavior. This approach is promising for monitoring model strategies while learning and allows to quickly spot model weaknesses. Prototypes further help to validate newly seen predictions by comparing them to prototypes, making it easier to identify outliers or assign predictions to known model strategies.
Speaker Bio:
Maximilian Dreyer is a PhD student in the Explainable AI group at the Fraunhofer Heinrich Hertz Institute in Berlin, under the guidance of Sebastian Lapuschkin and Wojciech Samek. His research centers on creating concept-based XAI methods that are both insightful and easy to use, as well as developing frameworks that enhance safety and robustness of AI models using XAI insights. https://www.linkedin.com/in/maximilian-dreyer/
16. Concept-based Explanations for Large Language Models (English)
Reduan Achtibat (Ph.D. Student, Fraunhofer HHI)
Abstract:
Large Language Models (LLMs) present a significant challenge for Explainable AI (XAI) due to their immense size and complexity. Their sheer scale not only makes them expensive to run and explain but also complicates our ability to fully understand how their components interact. In this talk, we introduce a highly efficient attribution method based on Layer-wise Relevance Propagation that allows us to trace the most important components in these models. Additionally, we can identify which concepts dominate in the residual stream and use this knowledge to influence the generation process. While this is a promising first step, there is still much work ahead to make LLMs more transparent and controllable.
Speaker Bio:
Reduan Achtibat is a PhD student specializing in explainable AI at HHI Fraunhofer Berlin under the guidance of Sebastian Lapuschkin and Wojciech Samek. His research focuses on making AI models safer, more transparent, and explainable. He is particularly interested in extending the current state of the art with concept-based explanations in the vision and language domain. https://www.linkedin.com/in/reduan-achtibat/